Genetic Innovation Requires Leadership and Data
The phenotype paradox in the beef industry.
March 18, 2025
Birthweight and weaning weight records were once considered innovative genetic improvement. Those were the relatively easy traits, and as decades passed more complicated areas have been tackled from fertility to incorporating genomics.
Today, beef industry leaders are looking at the next chapter in genetic improvement.
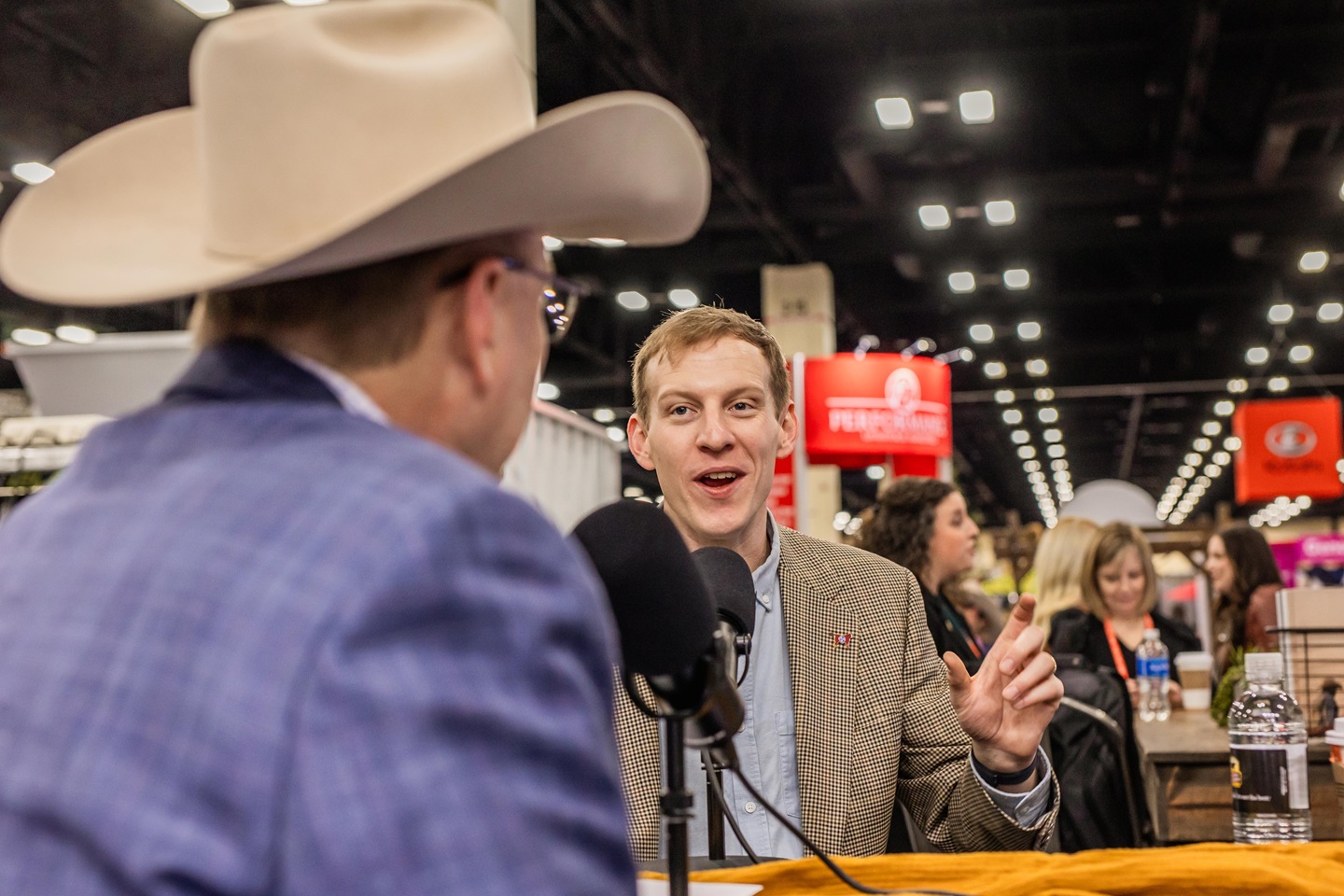
"Phenotypes are the currency with which genetic evaluations happen. The more phenotypes, the better your EPD is at the end of the day," says Troy Rowan.
“The more phenotypes, the better your EPD (expected progeny difference) is at the end of the day, and we’re really, really good at measuring easy stuff to measure,” said Troy Rowan, geneticist at the University of Tennessee– Knoxville. “The low-hanging fruit has been picked.”
He and John Dickinson, a fifth-generation Angus breeder and current American Angus Association board member, joined The Angus Conversation and covered the future of phenotype collection and using technology to make genetic improvement.
Traits with high economic value, from disease tolerance to fertility, often have the hardest to collect phenotypes, Rowan said.
“The more expensive, more difficult the trait is to measure, the lower the record number is, and the less likely we’re going to be to go out and collect those sort of pro bono as a seedstock producer,” he said. “Moving forward, how do you pull up the side of that line that’s on the difficult to measure, expensive to measure side and get a sufficient number of records?”
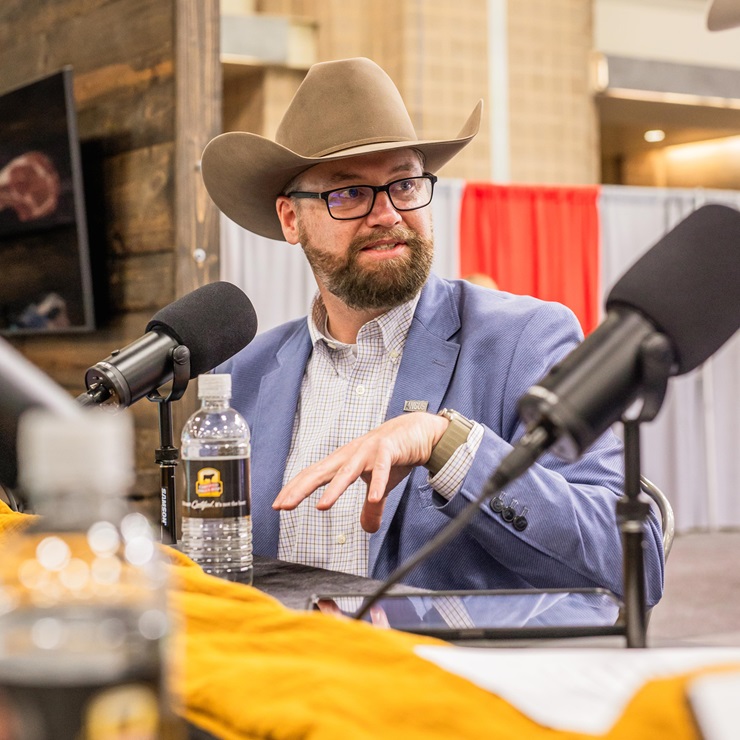
John Dickinson
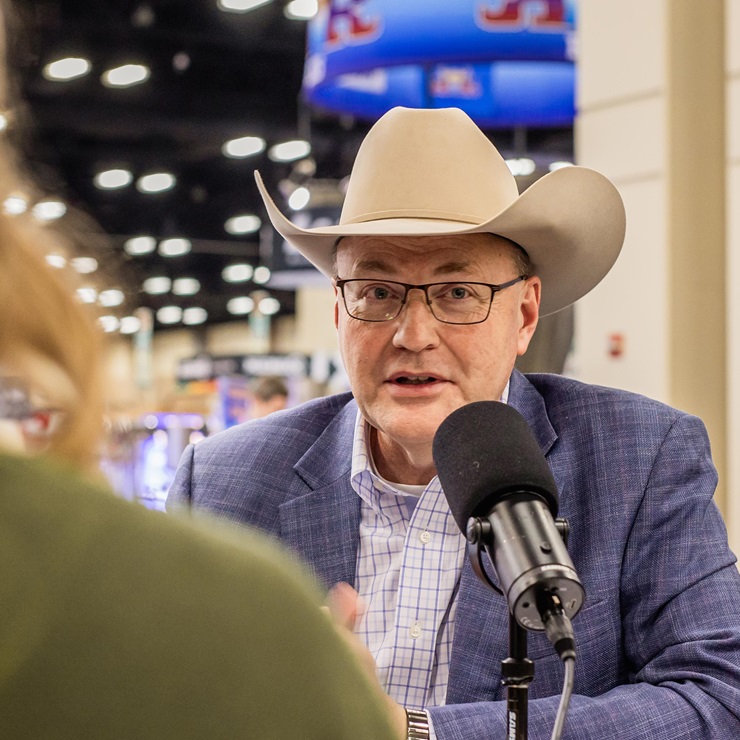
Mark McCully
Dickinson says he was energized by all of the ideas shared at the AGI (Angus Genetics Inc.) Imagine Forum, held in Kansas City, last fall. They’re summarized in the white paper Rowan authored from the event, “Exploring Next-Generation Phenotyping that Drives Commercial Profitability.”
“It was eye-opening for me as far as what the possibilities were,” Dickinson said. “The technology thing is moving increasingly fast.
Partnerships and data from nontraditional sources might drive improvements in the future.
“Looking at where this information is going to come from and what kind of power it’s going to have, I think there was a pretty good tone over this conference that commercial data is going to be a pretty large source of where to get it, how to get it,” Dickinson said.
To hear about new technologies like computer vision and microbiome analysis and the challenges of more data, such as labor and expense of proposing new traits, find The Angus Conversation anywhere you get your podcasts or visit this link.
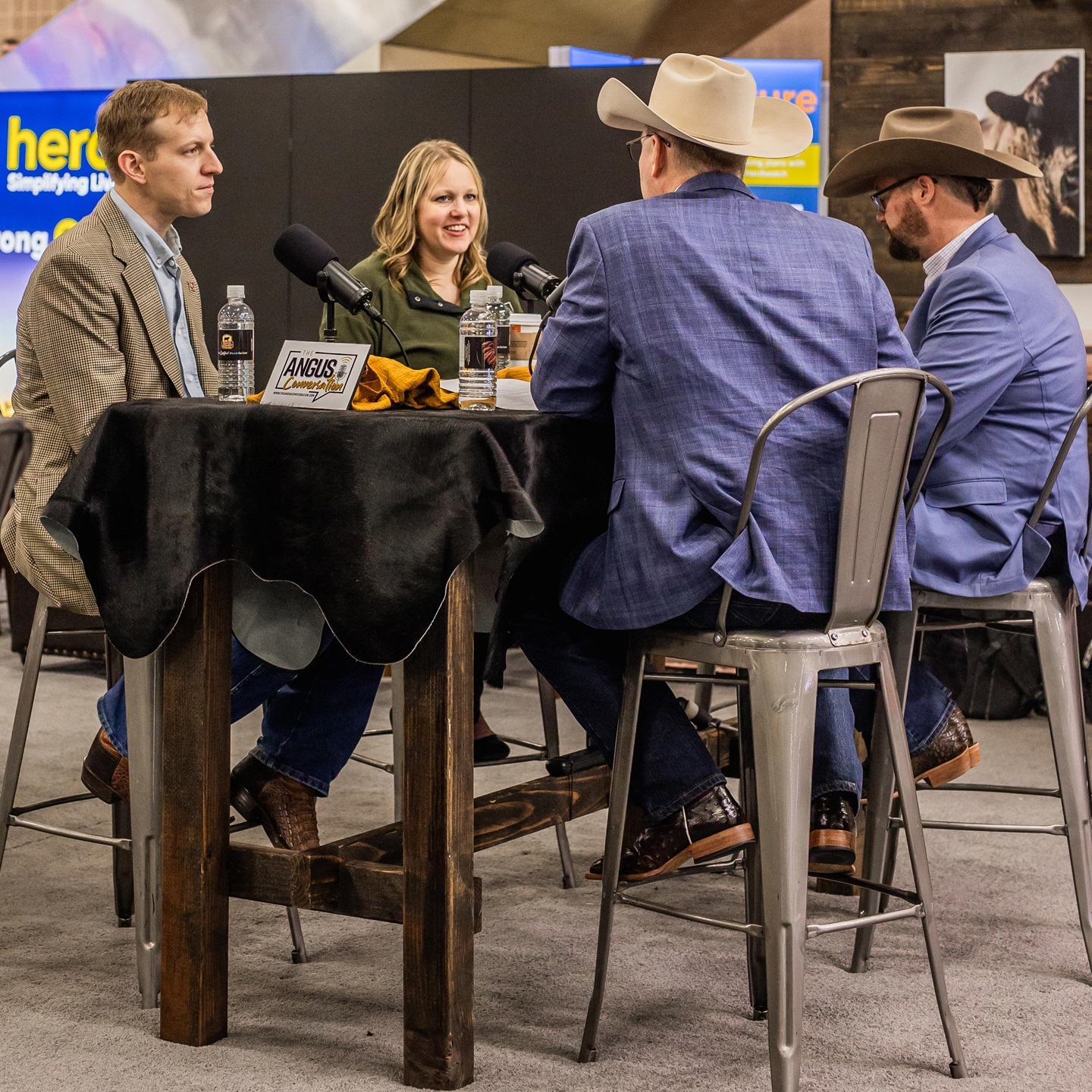
EPISODE NAME: Genetic Innovation Requires Leadership and Data — The Phenotype Paradox in the Beef Industry
Genetic innovation in animal agriculture is moving at a faster-than-ever pace, and this episode digs into the history of performance testing while exploring new technologies like computer vision, microbiome analysis and ways to collect more commercial phenotypes. The discussion covers the challenges of more data, including the labor and expense of proposing new traits, along with the opportunities to make big moves in important categories such as health and efficiency. Open-mindedness, collaboration, creativity and standardization will all be needed to make progress. What is the role of the American Angus Association and Angus Genetics Inc. (AGI) in leading this change? Listen to find out.
HOSTS: Miranda Reiman and Mark McCully
GUESTS: John Dickinson and Troy Rowan
John Dickinson is a fifth-generation Angus breeder and a former National Junior Angus Board (NJAB) chairman. A University of Illinois graduate and former regional manager for the American Angus Association, Dickinson often makes the trek between his cows in California and his home in Idaho. He is also a founding partner in Parnell Dickinson, Inc., a full-service marketing firm that works in all facets of promotion, management and cattle trade — both seedstock and commercial.
Troy Rowan is an assistant professor of beef cattle genomics at the University of Tennessee– Knoxville. His research work brings together many types of “big data,” both phenotypic and genomic, to understand the genetics of economically relevant traits in beef cattle. He also has a part-time Extension appointment, making all that research applicable to the state’s beef producers.
Troy grew up on a small Charolais operation in Southwest Iowa and received his undergraduate degree in biology from Creighton University. He earned his doctorate in genetics from the University of Missouri before coming to Tennessee in 2021.
RELATED CONTENT:
Exploring Next-Generation Phenotyping that Drives Commercial Profitability
Miranda Reiman (00:02):
Welcome to the Angus Conversation. I'm your host Miranda Reiman with my co-host, CEO of the American Angus Association, Mark McCully. And today we are going to kind of stretch you a little bit, Mark in this podcast.
Mark McCully (00:16):
This one is a little bit down the road, right? It's so easy to think about things that are right in front of us, tools and data that we have at our fingertips today. This session is really about trying to get the crystal ball out, trying to look around the corners and down the horizon to see what likely is going to be at our fingertips, maybe five and 10 years from now in the way of data and important data that might fuel our genetic evaluation and help us make cattle better.
Miranda Reiman (00:46):
So I think you've got to kind take off your, I guess, any natural barriers that say, we can't do that, or that sounds really hard, or how would we just take that all out and get a little bit creative as you listen to this one or maybe get a little bit, open-minded maybe,
Mark McCully (01:01):
Yeah, this one might be a little bit out there for some when we think about what could be, but I always challenge folks think about what you would've said wasn't possible 10 years ago or 20 years ago, and think about what we're doing today. And had we talked about that 20 years ago, you'd have said, no way could I carry this thing in my pocket that does all the things a smartphone does today. So I always try to keep myself in check when we think about some of these futuristic topics and what could be, and force ourselves to be open-minded,
Miranda Reiman (01:33):
Starting to feel a little bit like an old lady because I've started saying, well, when I first started, and then I go back to the way it was way back when. So I think that this will be a fun one no matter where you're at in the, if you've been in this industry five years or you've been in this industry your whole life, to really get excited about the future. Today on the podcast, we've got a couple of great minds in the genetics space. We are joined by Dr. Troy Rowan from the University of Tennessee Knoxville. Glad to have you on.
Troy Rowan (02:05):
Yeah, thanks. Glad to be here.
Miranda Reiman (02:07):
We've gotten to know you pretty well through the Beef Improvement Federation conference and I guess other genetic space initiative kind of things. You're kind of one of the up and comers in the genetics world, I guess I'd say, but give us a little of your background.
Troy Rowan (02:19):
Yeah, yeah. I grew up just about an hour north of St. Joe on a little Charolais operation just on the Iowa side of the border. My family's been in southwest Iowa for a hundred plus years. I thought I wanted to be a human medical doctor and went to Creighton University in Omaha, ended up back in the cattle business, did my PhD work at the University of Missouri and have been at Tennessee for the last four years and change.
Mark McCully (02:42):
When did the switch flip from the medical doctor field to PhD animal breeding geneticist?
Troy Rowan (02:49):
Yeah, there's this little thing called organic chemistry that comes for all of us. It changed everybody. It changes everybody's mind. So that was a couple years in and I realized that it just wasn't for me, and I really, really missed the cows and that impact, and it was the best D I ever got.
Mark McCully (03:08):
And your role today is extension teaching research?
Troy Rowan (03:11):
Yeah. I've got a 70% research, 30% extension, but a lot of freedom. I sort of do what makes sense for the industry. Those things bleed together and hopefully we're doing research that impacts producers in an applied way and vice versa. That extension, I think, delivers research, hopefully back to cow-calf operations and seedstock producers. So try and blend those things together as best we can.
Miranda Reiman (03:35):
I'll brag on Troy a little bit. Journalists always look for the really smart people that can explain it to people like myself. And so if you've ever got genetics questions, he's a pretty prolific writer and puts a lot of things out and is interviewed quite often because he's very good at that. So if you need to understand something in the genetics space, Google his name and you'll find a lot of good stuff. And then across from me here, I've got John Dickinson, producer out in the West, Angus roots, and you're on our American Angus Association Board, and also the Angus Genetics Inc. Board chair.
John Dickinson (04:07):
Yeah. Yeah, it's been a great trip on the board. I did grow up in the Midwest as well and moved out to California about 25 years ago, been out there ever since. Recently, the last, I guess, four years. I lived in Idaho, but my cattle are in California and we've got a marketing company and growing our herd. I was fortunate enough to get on the Angus Board in 21, and it's been a great experience. Angus Genetics Incorporated, just to watch that company grow in this time is great. I'm excited about being the chair in that organization this year.
Mark McCully (04:39):
And for our listeners that don't know how that happens, it was elected by your peers back in, in the November board meeting afterwards where the subsidiaries are established and chairs of those subsidiaries are voted on by your board members. And so congratulations and excited to have you at the helm.
John Dickinson (04:55):
Yeah,
Mark McCully (04:56):
Yeah.
Miranda Reiman (04:57):
Excellent. Well, I guess let's just jump right into our topic today. Mark. I wasn't at the AGI Imagine forum, so maybe you can set the stage just a little bit with what we're kind of recapping now. We're talking about what the fruits of that event that happened last fall are coming to be.
Mark McCully (05:13):
And I'll do my best Kelli Retallick Riley impression because really Kelli, I want to give Kelli all the credit in the world as our president of AGI, this vision around hosting an event. I think one of the topics, and we've called it kind of alternative data or non-traditional data that's been on the boardroom, that's been on the minds of staff and academics for some time. This whole idea of what are the phenotypes we all understand, the phenotypes are the key to the genetic evaluation. We love genomics, but it always comes back to the phenotypes. So what are the phenotypes that we know today like birth weight and weaning weight, and I don't want to say simple things to measure, but simpler things to measure traditional,
(05:55):
But then what's next? Are we going to be able to describe of genetic contributions to traits like animal health and disease resistance or bovine congestive heart failure? And then you start thinking about all of the new, and we're sitting here at the trade show, and if you walked around this trade show, there's lots of new technologies and innovations and things coming online and big data and AI and all this stuff. So it was really the impetus of this conference was to bring a group, really a cross section group together of breeders, of academics, of researchers, allied industry partners, a relatively small group. Gosh, I don't know, we had, what, 70 some people in the room. Something like that kind of evenly distributed. And let's get really smart people together, throw out some really big ideas and kind of vision what the future of phenotyping is going to look like, and then how do we kind of best position to get ourselves in line for that. So we had that and then tasked you, Dr. Rowan with OK, summarize all the things that were talked about in a white paper and really we're really excited here really this week and to be launching that white paper.
Miranda Reiman (07:15):
Yeah, absolutely. I think it walked through really logically, and I kind of just cheated. I took the white paper, and I think we're going to talk about it a little bit in that kind of bulleted fashion, but as any good white paper does, we started with, you started with setting the stage or giving us kind of the background of the start of performance programs and what traits were collected in the beginning. And so maybe walk us through a little bit of that, either one of you.
Troy Rowan (07:38):
Yeah. Yeah. I guess we started, Brian McCulloh started talking about the history of performance testing in Angus. And the thing that stands out is this isn't ancient history. This is fairly recent in the grand scheme of things. We didn't start weighing our cattle until the 1960s in organized performance testing. We'd done visual selection of animals since domestication, right? Our one tool was sort of what our eyes told us, and then we realized, right, to ratchet up that selection accuracy, we've got to start measuring phenotypes and then EPDs come on and there's all of a sudden the machinery, try and isolate that genetics. But really, the history has been a slow incremental change in phenotypes and then a fairly recent explosion in the number of things that we understand have economic importance and that we really want to try and capture. It's been a pretty quick evolution over the grand scheme of things. I don't know if you'd add anything else.
John Dickinson (08:39):
Yeah, well, just to touch on what Mark said earlier too, I think the audience and crowd was just excellent. You've got the producer segment, both some seedstock, some of our members and commercial producers moving on to the allied industry type segment. Then the scientific segment, not often are all them in the same room talking at that kind of level. So I really appreciate that. And I guess we had some linchpin type people in the room too that could tie like Dr. Rowan, like Kelli, they could tie those people together and see what the needs are from this sector and what they can do and is that applicable over in this sector. And I appreciated that. I thought just having that I got an audience who had a lot of value to this conference as well.
Miranda Reiman (09:17):
Absolutely. As you heard Brian McCulloh talk, did you think, oh, yeah, I was around for some of that genetic change. What kind of change have you seen in your history with the Angus breed?
John Dickinson (09:27):
Yeah. Yeah, I guess you could see that. I mean, we've got a good foundation of our traditional measurements, and I think it probably goes right along with the way technology comes along. If you would've thought maybe back in the day they were talking about taking this machine and putting it over the back of a bull or a steer after they mowed some hair off of it and taking all this information, and that's going to be useful to give us some kind of carcass traits. It probably would've been a little bit
Miranda Reiman (09:51):
That was crazy talk
John Dickinson (09:52):
Crazy talk. But that became just a standardization and it was implemented properly where you figure out a standard way to take that measurement through the ultrasound guidelines and so forth and really utilize it. And I think that trait really propelled us in those traits of the time. I think this conference was there to maybe look and see what other relevant traits are. I mean, I think both. We talked about the cost side, we get all the output traits, but there's probably some cautions we need to look at. Back to your question about Brian McCulluh. Yeah, it seemed like when we started talking about it, I'm like, yeah, that was all part of the system and I think it's going to go faster. I think these technologies, and we're here at the NCBA convention today and hearing about the technology space, the way it's advancing from things on smartphones to apps, and when I saw some of the pieces with this computer vision, we'll probably get into in the conversation, I was a little blown away. I mean, it looked like that movie Terminator. I mean, we were scanning across this landscape and taking measurements, I mean, thousands of measurements in real time, and I didn't think that was possible. And yeah, can't be implemented. I'm not sure we'll chat about that as well. But
(11:02):
Yeah, it was a good, eye-opening for me from the producer segment. Now maybe in the scientific segment, they're used to all that kind of stuff. But yeah, it was cool.
Troy Rowan (11:12):
Yeah, it's very much stuff that has to this point lived in academic papers. The concepts have existed for a little bit, but this was the first time that I think I've sat down with a bunch of producers and industry folks and actually talked about how do we logically do this, right? This isn't just theoretical, this isn't in the Journal of Computer Vision. This is on the mind of seedstock producers, right? Commercial producers and folks all around the industry. So how do we actually make this thing work? So it was exciting to take that next step and start to actually think about what happens when the rubber meets the road of these technologies.
Mark McCully (11:53):
We have so much, and I talk about this all the time, this convergence of technologies, because I really think it's that important. I think it's not just that, oh, we have this camera that can do this thing now. We have the computing power to take the data. We have cloud-based storage, we can move the data. We have analytic tools that we can make sense out of. All of these things are coming together, and I think anytime we see that in any sort of industry, you see this rapid acceleration of innovation, and I think that's what this feels like to me right now as we think about where we're at in terms of the future of data. John, one of the things you mentioned is around standardization and of the data, and I don't want to ever miss how important that is, and maybe Troy speak to that a little bit in terms of maybe BIF's role, and I want to give BIF Beef Improvement Federation so much credit over the history of where we've come of being able to really be that guiding body and set some standards that allow us all to kind of collect in some of the same way, and how does that evolve into the future a little bit?
Troy Rowan (12:58):
Yeah. I think standardization both becomes easier with some of these technologies, and it presents a really, really big challenge as you have multiple platforms, multiple technologies, all aimed at measuring the same increasingly complex phenotypes. So I guess going back to computer vision, like we mentioned there earlier, that's a super powerful tool, but like you said, there's thousands of different points in context and times and backgrounds,
Miranda Reiman (13:27):
And which ones matter?
Troy Rowan (13:27):
Yeah. How do you tease the actually important information out of that really, really high dimensional data and do it in a way that's portable from farm to farm, from commercial operation to seedstock operation? We talk a lot about commercial data being important, that lowers the barriers of capturing it, but it presents all those challenges that come along with trying to put things on some sort of a level playing field. So data standardization, again, we can be objective with a camera score, maybe in a way that me scoring a foot versus Mark scoring foot looks a little bit different. Cameras can maybe do that objectively, but if you've got 10 different softwares that do it, they've got to come to some sort of an agreement and standardize that so that everything is portable across the evaluation
Miranda Reiman (14:14):
And economically easy for someone to implement too.
Troy Rowan (14:17):
Absolutely. Absolutely. Cost is is the biggest factor here. We can't, I guess, expect the full burden of phenotyping to keep falling on just our seedstock producers backs as this gets more expensive. We've got to think about economic ways of doing this. Some of this technology is fairly cheap, some of it is quite expensive, so it's got to make dollars and cents for our producers across the industry.
Mark McCully (14:43):
You had an illustration in the white paper, you called it the phenotyping paradox. I thought that was really kind of a neat way to look at, and I am ...we're in a podcast and I'm going to try to explain a graph, that's bad idea.
Miranda Reiman (14:55):
We'll link the white paper in the show notes, so you can go look at it right now if you want. While Mark's trying to describe it
Mark McCully (15:02):
I'm not going to try. I was going to turn over, to Troy and try to have him describe it
Miranda Reiman (15:05):
You describe it, all those arrows and pictures. Go for it, Troy. Yeah.
Troy Rowan (15:08):
Yeah. Podcasting is quite a good visual medium, right? See what I have here on my iPad? Yeah, no, the phenotyping paradox, I sort of use this as I talk about phenotypes are the currency with which genetic evaluations happen. The more phenotypes, the better your EPD is at the end of the day, and we're really, really good at measuring easy stuff to measure. The low-hanging fruit has been picked. We've got enough or not enough. We always want more birth weight records, calving ease records, weaning weight records. But as we get into some of these traits that we know have a concrete economic value, very important disease tolerance, more precise measures of fertility, those get more and more difficult to measure. And as a result, we've got far less records there. So the more expensive, more difficult the trait is to measure the lower the record number is, and the less likely we're going to be to go out and collect those sort of pro bono as a seedstock producer.
(16:06):
So the phenotyping paradox, I sort of sketch out a suite of traits that we know have economic importance, and it's a pretty non-linear drop off there. And Andre, as we were writing the white paper, actually pulled records from the Angus database and it nearly perfectly matches my little concept mock up there. The more difficult the trait, the less records that exist. So I guess moving forward, how do you pull up the side of that line that's on the difficult to measure, expensive to measure side and get a sufficient number of records to do some damage with an EPD? Yeah.
Miranda Reiman (16:42):
If you're using technology that maybe, I guess we heard this in a podcast we recorded yesterday that maybe you can fill in some of the holes of that. Can you have fewer records today and tell more because
Mark McCully (16:55):
You simulate phenotypes, really
Miranda Reiman (16:56):
Yeah, exactly. Because you're able to kind of fill in, I guess, for those gaps.
Troy Rowan (17:00):
Yeah, that's sort of an evolving space, trying to use existing really complex phenotypes. I've seen this mostly in the context of red meat yield, doing some,
Miranda Reiman (17:11):
That's exactly what we were talking about
Troy Rowan (17:12):
Exactly the context you were talking about. But, get these really, really hard, expensive to measure phenotypes, but then use these new statistical tools, machine learning, artificial intelligence algorithms to take lower, easier data to capture. So maybe a 3D scan of a carcass with an iPhone backed up with some
Miranda Reiman (17:33):
Kind of impute, it
Troy Rowan (17:34):
Kind of impute that carcass data from the CT scanner or whatever. And again, you're able to with a lower number of the really complex, expensive records, expand that out to a much larger set of animals with a cheaper method that you capture data on. So
Miranda Reiman (17:51):
Maybe, maybe,
Troy Rowan (17:51):
Maybe, maybe. Yeah, it's an option we didn't know was in the toolbox until fairly recently. Right?
Mark McCully (17:57):
Sure. A couple of things you mentioned Andre and I should have. I failed to really give recognition also to Dr. Andre Garcia on the AGI team. He was also very instrumental in setting up this conference and working with you to help compile the white paper. So again, shout out to Andre, such a talented guy. We're so thankful he's on our team. John, when we think about all of this discussion around phenotyping with your cattle producer hat, with your board member hat, I know one of the things we hear from time to time from members and breeders is like sheesh, that's all you guys talk about is wanting more data, more data, more data and data
Miranda Reiman (18:34):
You sit in St. Joe and dream up new things for us,
Mark McCully (18:36):
Just come up with new ideas. So how do you defend what we're even talking about here?
John Dickinson (18:40):
Well, I think that the whole aspect of this topic where we're talking about technology and we're talking about the possibilities, I think that also applies to a lot of data we're collecting already. You mentioned like the foot scores or maybe that's an easier way to get a hair shed. Or maybe there's a lot of producers out there that have a hard time getting mature height, mature weight on their cow herd and so forth. If they've got computer vision and can run those cows into the lot and they've got a repeatable record, do it year after year and they've never had those mature weight or mature height or those kind of numbers. I think that's very applicable to what we're talking about. And I think for the essence of some of those really hard talking about those disease resistance or feed efficiency or some of those kind of, we haven't had those numbers or that database, just that they're hard to get. This is kind of showing us the opportunity of what we can do going forward. I think there's a lot of folks that are interested in those traits. They don't have a device in their pasture to measure those traits. And yeah, I think it's pretty exciting about that.
Mark McCully (19:41):
And they're economically relevant, and I think you touched on the right at the very beginning. We're not talking about novelty
Miranda Reiman (19:46):
Not just because we get interested in that
Mark McCully (19:47):
...just because we can, these things obviously have to be grounded in economic relevance, if they're not economic relevance, we probably shouldn't be wasting our time. Now, all of that to say there may be some things that we find, some phenotypes that we collect that we don't know they're economically relevant until down the road. So it's one of those things, it's probably from a geneticist standpoint, I know we hear it with our team, collect it all. We'll figure it out later, but so we just always be mindful of that.
John Dickinson (20:15):
And I think in the paper, Troy did a nice job of breaking down those four categories of what are the useful information that are easy to get? What is the useful information that's kind of hard to get. Then on the flip side, maybe the information that's not so useful, that's easy to get. We're not looking at those as far as a priority as far as going forward. Yeah,
Mark McCully (20:33):
That's a good litmus.
Miranda Reiman (20:35):
We heard yesterday talking to some cattle feeders when we asked what their main message was to Angus breeders. I mean, they said that sort of resilience and health suite. So again, it's things that the industry is asking us to improve in our cattle that we know could get assigned economic value.
Mark McCully (20:52):
And I guess maybe just inherent in our industry, we love our independence. That is cattlemen, right? So versus some other industries, competing proteins where things are vertically integrated, that data that's being collected more easily obviously gets shared, gets maybe the strategy behind their data collection is much more clear versus in our business where this industry, there's a lot of data being collected, but it's not being aggregated very well. And I know that's a big discussion of the AGI board of what role can Angus and AGI play in that area, because ultimately what we're trying to do is make sense of the data, put it into something that's valuable the cattlemen can use to advance the industry. So that's another inherent challenge in our business is how do we make this data useful because there's going to have to be some sharing involved.
John Dickinson (21:49):
Absolutely. I think that was, we talked about AGI specifically and looking at where this information is going to come from and what kind of power it's going to have. I think there was a pretty good tone over this conference that commercial data is going to be a pretty large source of where to get it, how to get it, and I think breeding records, for example, I think that's a great example of how we can become a partner or AGI, being a standalone company has the ability to go out there and serve that contract and get the information, bring it back into Angus, and provide that commercial producer or that side of the industry. We're probably at a threshold, I would guess, from seedstock information on breeding records.
Mark McCully (22:30):
Well, and just some challenges of, and I had this discussion with the breeder just last week that was a little concerned, and we hear from time to time, as quick as we're trying to turn generations, how do we know what those 8, 10, 12, 14-year-old cows, I mean, yes, we need more data because in a commercial setting, as long as she's bred, she's probably going to stay. But in a purebred and registered setting, you may be turning those generations far faster. And so how do we make sure we're able to have a full look of that female and be able to maybe get at some longevity things a little better?
Miranda Reiman (23:06):
When we're talking about collecting data from multiple different sources and getting some of that commercial data and things like that. Are there new technologies that will make it easier to bring that into the database? I mean, again, when you talk about the standardization, and I know that having clean data is super important to these projects,
Troy Rowan (23:22):
And I think to go back to something John said, some of these things are even easy for us to measure now, but the act of reporting is maybe the big hurdle for folks. I can capture a hair score, but is it just another thing for me to go in and input right?
Miranda Reiman (23:39):
More time in the office?
Troy Rowan (23:40):
Yeah. So much of this, I think is both more precise ways of measuring things in a standardized manner, but also if we can use technology to automate that reporting process so that it's a straight data flow from a mounted camera into Angus's phenotypic database, that's a win-win for everybody. The standardization happens and in real time, and it shows up and it shows up, and I don't have to do 10 clicks in a couple enters of numbers. I think it helps all around the more technology we can pull in here, you're not only measuring new stuff, but you're making that measurement and reporting more automated, which is another huge benefit.
Miranda Reiman (24:23):
Well, and especially as we're reporting data every Friday to our members, can you shorten that time so it doesn't sit in somebody's desk for a month and a half before they have time to input it?
Troy Rowan (24:33):
Right.
Mark McCully (24:33):
Absolutely.
Troy Rowan (24:34):
That's really cool.
Mark McCully (24:35):
Maybe let's dig into some of these technologies. In the white paper you really outline. The first one was computer vision, and we kind of heard some different presentations and discussion around computer vision. So maybe tee us up there, Troy.
Troy Rowan (24:48):
Yeah, computer vision I think is the one that really excites me a lot because we're in a lot of sense not talking about tens of thousands of dollars of hardware. These in a lot of cases are webcams. They cost,
Miranda Reiman (25:02):
That excites you as a producer too, right?
Troy Rowan (25:05):
20 or 30 bucks for five webcams and you plug them in maybe a high traffic area and you're able to capture, John mentioned repeated body condition scores, estimates of mature weight, mature height, and these are repeated records. So algorithms on the backside can take that image of a cow once, twice, three times a day and start to estimate those with pretty good reliability. And again, if these are connected, I think somebody mentioned the starlink has been a big deal, being able to have internet connectivity in remote places. This phenotype data can get captured, processed, onsite and then beamed to St. Joe and into your guys' database, which I think is enormously exciting. So lots of opportunities to use that camera data with fairly low barriers to entry. But again, the tough part is how do you build the infrastructure to process that data, send that data back to Angus, and then enter that into the evaluation. There's some challenges, but the technologies there and the hardware is not terribly expensive.
John Dickinson (26:13):
And just really quick, I mean just maybe also describe what computer vision is for somebody that's never heard it. Never seen it. That was my first exposure to it, and I thought it was like I said, something out of a sci-fi movie, that software that's applied to that camera and just finding all those points or taking points off the animal to,
Troy Rowan (26:32):
Right, right. Yeah. I think a lot of the software that gets built behind these technologies is it's looking for focal points on an animal. So it's just video data being streamed in real time, but these artificial intelligence algorithms can pick out, this is 10 points on the head, this is the 10 points on the back line, the 10 points that we know that this is indicative of structural soundness. Maybe if these two points are moving in this relation to one another. So again, the data depth is so huge. There's so many points, and again, this is video data, so over the course of time, it's really, really high dimensional, but the amount of information we can glean from that is extraordinary.
John Dickinson (27:19):
It's also IDing those cattle through the structure, right?
Troy Rowan (27:22):
Yeah. The head structure and eye setting and can with even on completely black cattle, it's fairly easy on a Holstein cow to pick out those face patterns. But again, it's just like a fingerprint for us or a retina scan. Those are,
Mark McCully (27:39):
Or my iPhone.
Troy Rowan (27:40):
Exactly.
Miranda Reiman (27:41):
I'll get my phone and it unlocks.
Troy Rowan (27:43):
Yeah, and the same thing is possible for cattle, which from an ID standpoint, you're not reliant on a tag that maybe falls out to tie that record able to use those algorithms to understand which cow is doing what, where and when.
Mark McCully (27:58):
Yeah. Well, I think the quote and it was captured in the paper, an image is worth a thousand measurements and maybe more, right? It might be worth tens of thousands of measurements though. It's a different way of thinking about phenotype and all the different measurements over time.
Miranda Reiman (28:17):
We are going to take a quick timeout for a word from Angus Media. To make good decisions, you must be informed. At the Angus Journal, we have you covered. Subscribe to our monthly magazine to be part of the direction of the Association and influence decisions that will affect your business. For just $50 a year, you get a high-quality publication filled with good cattle and stories of good people delivered each month. Change is inevitable. Don't get left behind. Visit angusjournal.net to subscribe today. Now let's pick up where we left off.
(28:54):
John as a producer sitting there. I just heard Troy say, and then we'll beam up to St. Joe when you just said sci-fi. So I'm sure these are going to get selected as quotes from this podcast. Does that make you mostly excited? A little bit nervous, all excited, not nervous at all, or nervous and only a little bit excited? I'll give you multiple choice.
Mark McCully (29:13):
A spectrum.
Miranda Reiman (29:13):
Yeah, that's right.
John Dickinson (29:14):
I would say only nervous because I am not sure, one, what's it going to cost? And two, how is he,
Miranda Reiman (29:20):
He said 30 bucks, I think.
John Dickinson (29:22):
How could it be implemented and so forth. We talked about before we, we were able to use ultrasound technology and come along with that, we're able to punch DNA samples out of ears. So,
Miranda Reiman (29:35):
Which was scary when we first started that too, does this stuff work?
Mark McCully (29:37):
Think Star Wars.
John Dickinson (29:39):
And so I think once get the implementation down and it becomes useful, I think it can be a great opportunity. So I guess I'm not too scared about that, but I don't want to say concerned, but I mean, again, once you get all that information and that data, how do you use it? How do you standardize it? And again, are we going to go, this Imagine Conference was great for trying to get people thinking about what are the really good traits we want to get after?
(30:06):
I guess nobody left there thinking, well, we have computer vision now let's go just shoot video across the entire cow herd and see what we get back.
Miranda Reiman (30:13):
We need some strategy behind it.
John Dickinson (30:15):
It's good to bring those folks together and kind of target what are the traits that are of interest to us? What can we utilize best for our membership and for our genetic evaluation? And then, yeah, are these things like we talked before, they can also make foot scoring or other trait collection easier too. So I think folks are mean trait scoring is just we have a standard system. I talked to a lot of my fellow members and producers that it's kind of a struggle. Some guys that just don't like to do it because I have their son do it or I don't feel comfortable doing it, calling a five, six or seven or whatnot. So with this technology, I could help that too.
Mark McCully (30:48):
So Troy, help us think a little about, because one of the things I think I personally struggle with, but as an animal breeder, as you think about, I mean, our systems today are built on contemporary groups and ratios and differences within contemporary groups. That's how we build comparisons and use the phenotypes, and that's how we can compare a herd in Georgia to a herd in Montana. It's about the contemporary group. Talk about what does that require different models when we start thinking about incorporating these phenotypes or is technology going to solve the contemporary group situation by pulling out temperature and humidity and whatever? How do you think about that from a contemporary group? And is it going to require a different model for us to start building EPDs from?
Troy Rowan (31:32):
Yeah, I think that's a
Miranda Reiman (31:33):
Troy's like, give me a crystal ball Mark and I'll tell you
Troy Rowan (31:35):
No, I think that's a good question, right? Because the technology doesn't stop at our measurements of the animal's phenotype. I think it gives us an opportunity to dig into the environment at a scale that we probably didn't before. That camera that's capturing a cow's foot score and body condition score in real time can passively be looking at the forage availability behind her, a temperature sensor for another three bucks added onto that system, right? I mean,
Miranda Reiman (32:06):
$33 again.
Troy Rowan (32:07):
Yeah, three more dollars.
Mark McCully (32:10):
Because contemporary groups are, they're just trying to parse apart environment and genetics, right?
Troy Rowan (32:14):
Exactly. Exactly. And so the better handle we have on environment, and not just environment but management, which flows into another project that we're working on with Angus, right? But how do you account for that environment and model that more appropriately make that genetic selection tool at the end of the day more accurate? So I think it's a great question Mark that's going to evolve along with these technologies. Contemporary groups are still going to be the core of what we do for a while, but as we figure out different ways to model environment and how genetics interact with that environment, then yeah, there's a lot of opportunities for us in the future to look at that through a little bit more precision matching genetics environment and everything together.
Miranda Reiman (32:59):
So we've talked a lot about phenotypes that you can look at and see, or even maybe phenotypes that we can be measuring today but don't have time to. But what about those phenotypes that are a lot harder to get at? I'm thinking about disease resistance and you're not going to have a camera that's going to be able to scan to decide who's less susceptible to BRD
Mark McCully (33:19):
Or which ones have temperatures.
Miranda Reiman (33:21):
I wasn't at the conference, I guess you're right. Talk a little bit about the opportunities maybe that exist in some of those that what are we targeting? What are we even looking at? Is it
Troy Rowan (33:32):
Yeah. I think that disease resistance tolerance, robustness, talk to anybody, again, whether they're at the cow-calf level, the stocker level, the feedlot level, and disease is going to be the missing piece that they're interested in. Unfortunately, it's one of the toughest ones for us to get a handle on because those traits tend to be more lowly heritable, right? Environments are so different. The pathogen in one place that causes BRD is going to be completely different than the pathogen in another place, which makes disease resistance, maybe different traits between those two. So that's a really, really hard one. And so I think we're still figuring out what's the standardized phenotype that we use to collect? Is it a way of measuring the intensity of an immune response in a standardized way? Is it using feedlot records from these commercial animals, getting treatment records on tens of thousands of animals and trying to build that way, some genetic measure of disease resistance. So I think there's lots of opportunities, lots of ways we can come at it. Maybe it is a bunk line camera that has thermal imaging and you can pick some of those things out that snotty nose, that temperature in the ear or something. There's lots of opportunities, but still a little ways off, and we've got some work to do on the disease front, but we understand the economic importance and animal welfare importance and all of that. Sure.
Miranda Reiman (35:04):
You had talked in there a little bit about how, for example, the dairy industry uses a indicator trait, so it has been done in other industries. When we look outside of beef
Troy Rowan (35:15):
For sure, no dairy, they use their routine milk samples to estimate a whole variety of traits. Cow metabolic status, mastitis, they're doing methane emission estimates from spectral imaging data of milk samples. We don't get very many milk samples on our cows, unfortunately
Mark McCully (35:32):
I think our breeders would not
Miranda Reiman (35:35):
Yeah, that's right.
Mark McCully (35:36):
So every morning we need you to go out, go out and
Troy Rowan (35:39):
collect a milk sample and send it to Troy
Miranda Reiman (35:42):
Well tell him John authorized it.
Troy Rowan (35:43):
Yeah. Yeah. But no, I think that finding indicator traits, whatever they are, especially if they're easy for us to capture, those are what all this is built on, right? Indicator traits have helped us. You've mentioned John ultrasound. Again, it's not the same as a real carcass record, but good enough. It gives us a glimpse on those animals that aren't terminal and can build the database to make a useful tool at the end of the day. So as we search and new technologies come online, finding those indicator traits and understanding where and how they're useful for traits that we thought were impossible to capture, those are going to be going to be great tools for us. Sure.
Mark McCully (36:28):
How about the microbiome topic? I'll get over my skis real quick when I start talking microbiome, but from some of the feedback of participants that were there, that was one of the topics that maybe surprised as many people or maybe they saw some potential that hadn't even been considered. So maybe help us with what that microbiome discussion was about.
Troy Rowan (36:53):
The microbiome is a little out of my area of expertise as well. But again, I think that it's important that we consider cows themselves as a bigger system. It's not the cow that's turning that grass into energy. It's those microorganisms that live in her rumen that allow her to convert that low quality forage into energy. And so there's variation in that microbiome, and part of that, of course is environmental, and part of that is actually heritable. There are some microbes that there are pretty appreciable heritabilities for that play important roles in things like disease resistance, feed efficiency, methane emission, all of these traits that we're interested in understanding. All of a sudden there's an added layer of complexity there. It's not just the cow, it's not just her environment, but that rumen microbiome, much of that is established early on in a calf's life. The environment, environmental stress diet will play impacts on the composition of that. But being able to capture that information and model it alongside genetics, I think can help us make more accurate phenotypic predictions on animals. So your accounting maybe for some of that environmental variation, if you can get a handle on the composition of the room and microbiome. So what bacteria is at a higher frequency. It's a really exciting area that we're still getting a handle on in a really basic way.
(38:22):
Most of those microbes haven't ever been cultured in the lab before. The rumen is the place that those exist on earth. And so trying to understand not just what's there
Miranda Reiman (38:32):
That's messy work
Troy Rowan (38:32):
But what does it do? What does it do exactly. Yeah. So it's really, really exciting in understanding how it plugs into genetic evaluation and precision management of animals eventually, tons of potential. But again, we're still in early stages and trying to catalog everything and figure out what's useful, what's manipulatable, and what do we do with this in the future. Sure.
Mark McCully (38:56):
When you think about that topic, and I mean, again, I'll get over my skis quickly, but I mean, I think one of the things we are always challenged with when we start talking about efficiency and identifying the most efficient cows, we don't measure feed intake on cows. So we have some assumptions based on body type on mature size, on pounds of calf weaned for the weight of the cow and all those. But in reality, we know there's a fair amount of variation probably within all of those, even body types we think are the super efficient cows. So in my mind goes to, is there a way, and you were talking about maybe even taking fecal samples over time, maybe on an annual basis, that you could start characterizing how different those cows potentially are. I mean, is that potential where this thing could go?
Troy Rowan (39:47):
Yeah. One of my research program's biggest interests is cow efficiency and how do you measure that? And again, our indicator right now is mature weight, but data is starting to show from various angles that sometimes those bigger cows are actually pretty thrifty. They can exist on a lower plane of nutrition than some of the smaller cows. So trying to understand and use these new novel technologies, whether it's the microbiome taking a fecal sample and estimating some level of metabolic efficiency, whether it's an ear tag based monitoring system that says, how much time does this cow spend grazing? I think there's lots of opportunities for us to get a better handle on how that forage based cow turns grass into weaned calf pounds. Again, to me, one of the biggest gaps that we have in our understanding of efficiency and profitability at the commercial level is how efficiently does that cow utilize my limited forage resources?
Mark McCully (40:49):
Who funds this research? I mean, when I think about one of the challenges, candidly, I believe in our business of we've got so much research that we need to advance, and the research model and research institutions is different. We have a lot of research going on in private companies and things. I guess, concerns? Am I the only one with that concern? Or how do we fund this really, really important research as an industry that we need?
Troy Rowan (41:17):
Yeah, at the present moment, most of this research is federally funded. The US Department of Agriculture has invested an enormous amount and foundational some really basic research that we need to do to understand these concepts and also applied research. So USDA is our major funder. There are a handful of other federal funding agencies that touch some of these topics. But increasingly, we're seeing that industry partners are important in seeing value in this research and helping us out, even if it's data sometimes, right? It's things that on a university farm, I don't have the capacity with my few hundred cows to get, but the power of a breed association or some large breeders with a shared vision can really help move some of these things forward if they've, again, share your vision and the importance for this. They're essential partners in doing this research and will continue to be more so as we look to the future.
Miranda Reiman (42:14):
So partners don't always mean dollars.
Troy Rowan (42:16):
Exactly. Exactly.
Mark McCully (42:18):
That's great perspective.
John Dickinson (42:20):
Yeah. I think as far as AGI goes, and with what Kelli has done there as president just an example of what she's done with the heart health initiative. I mean, identified a problem, identified a trait that had some concern or wanted more information on, and she went out and found the Five Rivers and pulled in other parts from Cargill to CAB, to other industry industry assets that would have interest in this kind of research, put a program together. I would assume this would kind of work the same way. And AGI has a great vehicle, I think to bridge those gap and have those connections. We start talking about trying to access that commercial data through our members and through their commercial bull buyers. And it's a great network. I think to somebody's going to have, when you go out and they ask is... We need this kind of information with this kind of management setting. We are going to have some members that say, yeah, I've got that opportunity right here.
Miranda Reiman (43:12):
I kind of wondered the same thing in the role of AGI. We've kind of talked about it here and there in getting that commercial data in and how do we, I mean, I guess we can all sit around here and talk about the greater good of the industry, but how do you get a commercial guy who's interested and yeah, I'll just collect all that data and give it to you guys. And what's the incentive there for the commercial industry to participate in some of our genetic programs
John Dickinson (43:35):
Sure. We start thinking about the tools that we can make, and you start talking about traits like better fertility through breeding rec. You start talking about getting way out there with the microbiome and having some kind of disease resistance. That's going to be a trait that's going to go across all sectors of the production chain. And I think that they'd be pretty interested in making some headway with that. But what we talk a lot about in the AGI boardroom, I mean, this entity is going to be a good partner and a good partnership. Both sides gets some kind of a win. So if it's something specific we can tag along with some other research, I think that's the opportunities that AGI is looking for going forward.
Miranda Reiman (44:13):
Absolutely.
Mark McCully (44:15):
We always kind of joke that if the commercial producer was really into collecting data and recordkeeping, they'd be in the seedstock business. I mean, they're busy doing a lot of other things, and so we think about how do you make it maybe more passive? And that's where I think some of the technologies kind of get exciting in my mind, of some passive data collection. And then I think you also, we've got to turn, we'll turn it into more powerful genetic selection tools. I think there's also some other things we could do, maybe maybe shorter term, maybe some benchmarking tools, some herd reports, some analysis that someone that's participating in this get some value back out of it, maybe more immediately even to identify, help them identify some places they can make more money. Ultimately why they would the short term, what's in it for them. I
Miranda Reiman (45:05):
Think about that in apps that I use or different things. I'll give away a lot of personal information for a more personalized experience. Or go through a lot of, take a quick survey and then I'll get what I need. So I guess you're exactly right that they'll be getting, hopefully a more personalized tool or something that they really need in their toolbox. Yeah, that makes a lot of sense.
Mark McCully (45:26):
As you guys look on the horizon, I mean, we threw out a lot of, are there some other technologies that came up in the discussion or other ideas that you
Miranda Reiman (45:36):
Or hallway discussions you want to air right now?
Troy Rowan (45:40):
Yeah, I think one of the ways, and especially as we consider how commercial data gets plugged into the system, the essential component to that, we've talked all about phenotypes, but commercial genomics is really going to be the thing that ties everything together. These animals that don't maybe live in the Angus pedigree, but their records are very valuable. So the link that's got to happen there is commercial genomics. How do we use that as an incentive possibly for commercial producers to contribute data and then use that genomic information to help them make better breeding decisions, better bull purchase decisions that are more tailored to reaching their selection objectives within herd. But at the end of the day, we've got to be able to tie everything together with genomics and that genomics can follow an animal and add value to that animal, not just at the cow-calf level, but through the backgrounding phase, through the feedlot, and ultimately on through harvest. So I think that the genomics, again, we talk all about phenotype, phenotypes are essential to making this work, but to pull that commercial information in, we've got to remember commercial genomics
Miranda Reiman (46:46):
And genomics will help you get to it quicker. Shorten that time.
Troy Rowan (46:50):
Absolutely.
John Dickinson (46:51):
And I think you touched on it briefly before. I mean, I think there's always been maybe some speculation about what is the environmental impact on some of our traits, or does it vary just because we've got a wide cross section of producers from Washington to Florida, and how do these traits, or did they all grow the same? Do they all develop the same or different environments based on environment? Like you said, we separated through the contemporary groups, but there's some opportunities here to maybe just to see what environment can play in most traits
Miranda Reiman (47:20):
As I look to the future. I wonder how you will balance the, I mean, you said earlier it's coming faster and faster and faster. Sort of the need to get these tools out there quickly and at the speed of business, but also make sure that they're accurate and well vetted. And I mean, there's kind of two competing. How are you going to do that, Troy?
Troy Rowan (47:41):
Yeah, that's a great question. No, I think that's going to be so important is understanding return on investment of these things. Just because we can measure something doesn't mean that it's economically important. And even if it's really easy to measure, probably it causes work at, in St. Joe, right? To make that into an EPD, there's got to be a really clear impact on the overall breeding objective, and it's got to move the needle in a way economically for our commercial customer. That's the goal of all of this. So again, you talked earlier about the importance of economically evaluating these traits, that's going to continue to be so important, and not just the economics of these traits as we try and fit them into the context of an index, but also the economics of measuring these things, right? If a trait moves the needle just a tiny bit, but costs $150 a head to measure, we probably would be better investing that money those times that resources in a trait that's easier to measure. So again, economics is going to be at the core of a lot of this as well as we try and prioritize what traits we measure on which animals, some might be better suited for a very targeted, specific population that samples a specific set of interconnected individuals. I think we need to be strategic about these more expensive phenotypes, whereas the easier to capture ones, we can do more of a blanket approach
Mark McCully (49:10):
As we think big picture. And maybe John, with your board member hat on, I mean, one of the things we talk a lot about is that at the advancement of all of this data collection and if you will, the potential silos of data, one of the things we talk a lot about is maintaining access for our members. And I think to me, that's why we're doing this. It's not that I think that for the greater good, but for our breeders, making sure that this data is accessible to them. I think if you look at the progress we've made in an industry, it's because cattlemen have had access to this information. They've had access to data. So trying to make sure we're positioned to keep that access flowing into the future as we get into some of these new technologies. I think that's a priority that comes from the boardroom.
John Dickinson (49:59):
Yeah, yeah. No, I would agree with that. I think we just need to look at one, when I saw all these new technologies and just from the videos, all I thought was this is going to take a lot of space. I mean, where is all this going to be stored and how is it going to be organized and those kinds of things. So infrastructurally, there was maybe a question I had. I'm not worried that we can't get that done, but then, yeah, how do we make that data interpretable and useful for the membership as well, I think is something we've always done with every other trait too.
Miranda Reiman (50:29):
So we've left with probably as many questions as we have answers, but that's maybe
Mark McCully (50:34):
Always, but that's kind of the purpose of that conference. Exactly. And I think I would like to say it's the purpose of the white paper is to really tee up some great conversations, some great questions, maybe open some eyes at times of some capabilities and some potential that exists out there in technology, stimulate more innovation. I think that was really the purpose,
Miranda Reiman (50:57):
And I was going to encourage anyone who's interested in this topic, definitely go read that white paper and then have those conversations on your own or call us up. Or really, like you said, it's supposed to be a starter to the topic. It's not like that was the
Mark McCully (51:10):
Ta-da
Miranda Reiman (51:11):
You closed it. Yeah, you solved all the problems and all the things of the future and close the book. So we look forward to more research, answering more questions, more partnerships, all the above, but really puts it together in a nice package. So before I ask the random question of the week, is there anything else that you guys want to add that we haven't talked about or any area that we haven't covered?
John Dickinson (51:34):
No, I just want to say I think it was a great program. I appreciated the widespread folks that were there. When you said eyeopening, it was eyeopening for me as far as what the possibilities were. The technology thing is moving increasingly fast, and I don't want use that to scare people that, I mean, I think it's probably the same way smartphones came along.
Miranda Reiman (51:54):
I mean, we feel that, we all feel,
John Dickinson (51:55):
We saw a graph the other day where smartphones just a couple years ago, they picked up more usage than any other technology piece we've had yet.
Miranda Reiman (52:03):
Absolutely.
Troy Rowan (52:04):
Yeah. No, and I think the couple big picture takeaways is the diverse group of people that were there is a testament to what it's going to take to actually make this happen, right? Yeah. This can't just happen in St. Joe, right? This can't just happen in the Angus breed. This can't just happen at the seedstock level. It's going to take real intentional partnerships to move the needle on all of this. But I think that the forum was a great way for us to jumpstart some of those conversations, start thinking at the level that it's going to take to address some of these challenges that we lay out in the white paper. But more than anything, it made me excited because I think it's possible when you get those sorts of people in the room together, we're going to make some progress on this in the near future, but it is going to take some pretty radical collaboration and outside the box thinking.
Mark McCully (52:54):
Yeah, absolutely.
Miranda Reiman (52:56):
All right. Random question of the week time. This was inspired by, we already know what Troy's maybe least favorite class in college was, but I want to know what your favorite class you've taken. You're thinking that was a long time ago... John?
John Dickinson (53:08):
I'm pretty sure it was. I am trying to remember the number. I want to say it was Horticulture 122.
Miranda Reiman (53:14):
Really?
John Dickinson (53:14):
Yeah. It was flower arranging for the home. I needed to
Mark McCully (53:18):
You use it every day?
Miranda Reiman (53:19):
I was just going to say,
John Dickinson (53:20):
I needed to fill up some hours, I believe, and I think I was one of three guys in a room of about a hundred, well, probably 80 or 90 people. And yeah, it was at a good time slot on a Thursday, I think, right around two or three clock Thursday afternoon. Yeah. Yeah.
Miranda Reiman (53:36):
You arranged some flowers and then picked up a date?
John Dickinson (53:39):
No, I mean, I can't say I went every day, but I got the A and the final was merely just an arrangement project. I actually, I lived at the fraternity house I lived in. I grabbed a vase on the way out the door and got some flowers on the way. I actually bought them but yeah. Yeah.
Miranda Reiman (53:59):
Well that is definitely not the answer I expected today. If you tell me basket weaving or waltzing or something, Troy
Troy Rowan (54:05):
No, I really can't top that. No. My favorite class is still atmospheric science. I had a great experience there. I took it on a whim and I don't remember a whole lot, but I can identify cumulo mammatus clouds whenever they come out. So still a little bit of useful stuff from that, but nowhere near a floral arrangement.
Miranda Reiman (54:25):
Alright, Mark, I'm going to make you answer too.
Mark McCully (54:27):
Yeah, probably the class I first thought of was an ag econ class by Dr. Drinka. Well,
Miranda Reiman (54:33):
No, what a predictable answer, Mark.
Mark McCully (54:35):
No, probably it was, he, one, and I'd say we could probably all say when you talk about a favorite class, it probably had to do with a favorite instructor. Right. But he made economics fun and exciting and probably opened my mind to economics. And I loved his. He had, the way he tested was you got a blank sheet of paper and it was write down everything you learned in the last, and it made you learn and retain a lot of things. And he was fantastic. He was very, very entertaining and fun. And so, yeah, I would say it was an ag econ class at Western Illinois University. How about you, Miranda? Every once in a, I get to remember to turn this question back on you
Miranda Reiman (55:16):
Yeah. Mine would be probably somewhat professor related. It would be advanced animal nutrition that I took with Dr. Hans Stein. And it was probably just because it was a really tough class for me. I got an animal science minor, so I didn't have to take all of the tough classes. I opted out of genetics actually. But it was a little bit of math, but applied math and I don't know, it was just, I could not balance a ration to save my life today. So don't ask me for any nutrition advice, but it was probably the process of figuring out something very complicated and saying, hey, I can do that.
Mark McCully (55:46):
Yeah.
Miranda Reiman (55:47):
I came out with an A. So that was
Mark McCully (55:49):
Yeah, that's important too.
Miranda Reiman (55:50):
Very good. That was. Well, thank you so much guys for being here for your contributions both to the conference and the white paper and your continued leadership in the AGI space and looking forward to what continues to come of this. And we'll probably have more conversations in the future.
Mark McCully (56:05):
Not to be overly dramatic about it, but I truly believe this is one of the most important things that we've come out of Angus in quite some time.
Miranda Reiman (56:12):
I've heard him say that before. He is not just saying that for your benefit,
Mark McCully (56:15):
I truly believe, I think this is a really, really important conversation, and I think that the conference and now this white paper, I just want to highly encourage our listeners, our breeders to dig in on this topic a little bit and learn a little more and ask questions and help us think about it. I think, Troy, what you identified so well is the technologies out there, the capabilities out there, the collaboration is going to have to be here for this to happen and play out the way we want it to for the benefit of the industry, benefit of our members. Most certainly. So appreciate both of you guys, your leadership and your talents, and we're excited to see where this is going to go.
John Dickinson (56:56):
Thanks for having us. Appreciate what you guys do as well.
Troy Rowan (56:59):
Yeah, thanks, this was a lot of fun.
Miranda Reiman (57:02):
I hope you enjoyed that one and a chance to stretch your mind as you think about the future. If you're enjoying this podcast and want to help other Angus breeders become part of the conversation, go rate it. Leave us a review on your favorite podcast platform, or share it with your friends. Thanks for listening. This has been The Angus Conversation, an Angus Journal podcast.
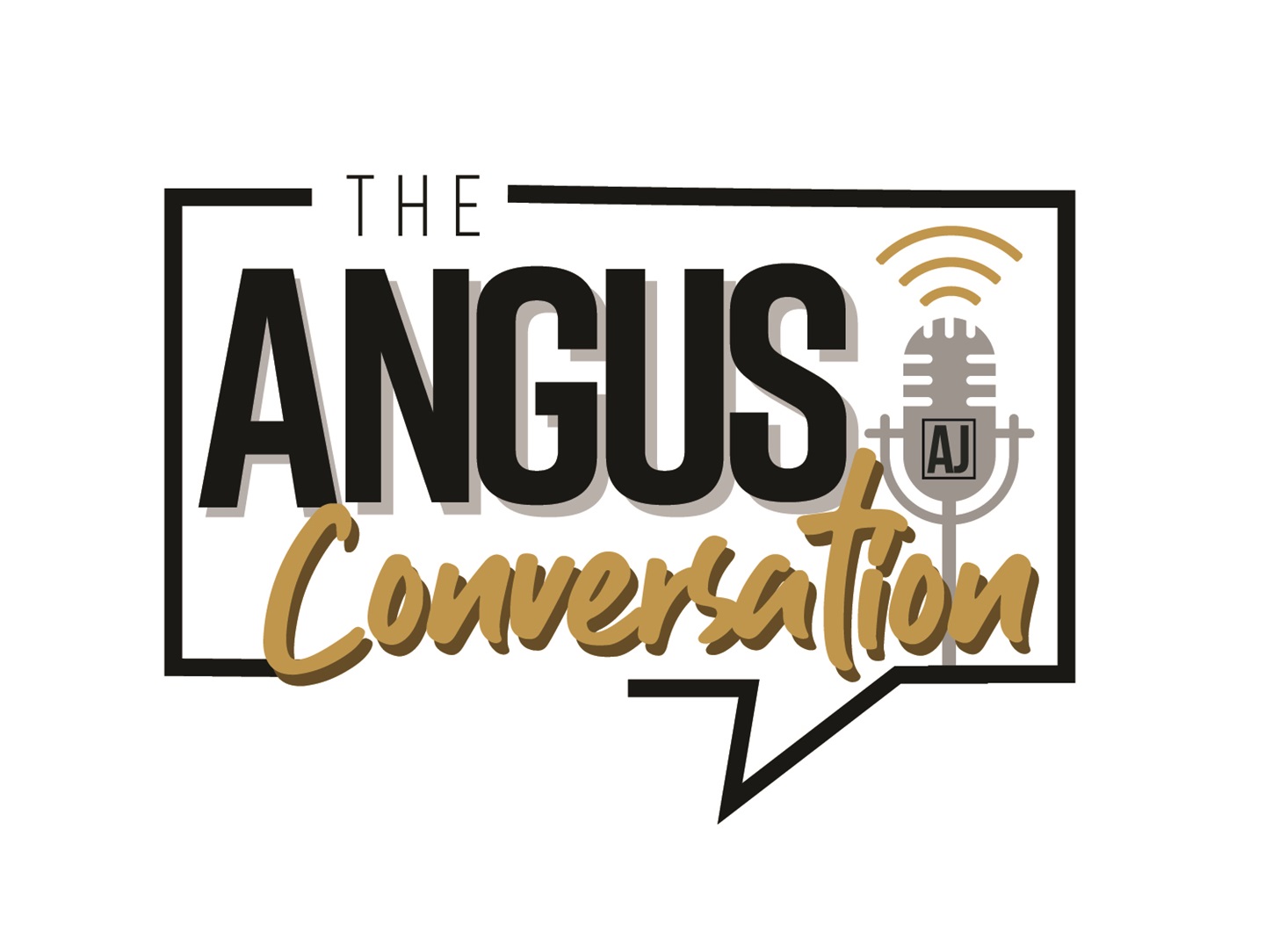